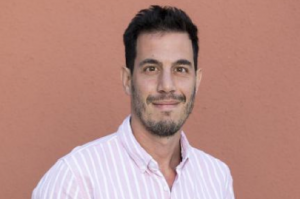
Manuele Leonelli
Assistant Professor
Inference over tails is usually performed by fitting an appropriate limiting distribution over observations that exceed a fixed threshold. However, the choice of such threshold is critical and can affect the inferential results. Extreme value mixture models have been defined to estimate the threshold using the full dataset and to give accurate tail estimates. Such models assume that the tail behavior is constant for all observations. However, the extreme behavior of financial returns often changes considerably in time and such changes occur by sudden shocks of the market. Here the extreme value mixture model class is extended to formally take into account distributional extreme change-points, by allowing for the presence of regime-dependent parameters modelling the tail of the distribution. This extension formally uses the full dataset to both estimate the thresholds and the extreme change-point locations, giving uncertainty measures for both quantities. Estimation of functions of interest in extreme value analyses is performed via MCMC algorithms. Our approach is evaluated through a series of simulations, applied to real data sets and assessed against competing approaches. Evidence demonstrates that the inclusion of different extreme regimes outperforms both static and dynamic competing approaches in financial applications.
Assistant Professor
INQUIRY -