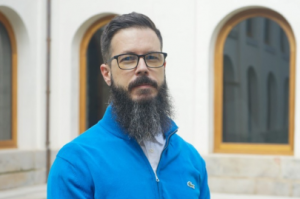
Miguel Vaquero
Assistant Professor
This article proposes a methodology for discretizing accelerated optimization flows while retaining their convergence properties. Inspired by the success of resource-aware control in developing efficient closed-loop feedback implementations on digital systems, we view the last sampled state of the system as the resource to be aware of. We illustrate our design methodology for discretization on a newly introduced continuous-time dynamics, the heavy-ball dynamics with displaced gradient. Our algorithm design employs techniques from resource-aware control that, in this context, have interesting parallelisms with the discrete-time implementation of optimization algorithms. These include derivative- and performance-based triggers to monitor the evolution of the Lyapunov function as a way of determining the stepsize, exploiting sampled information to enhance performance, and employing high-order holds using more accurate integrators of the original dynamics. Our approach gives rise to variable-stepsize discrete-time algorithms that retain by design the monotonically decreasing properties of the Lyapunov certificate of the continuous-time heavy-ball dynamics with displaced gradient.
Assistant Professor
INQUIRY -