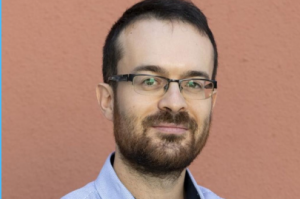
Rafael Ballester-Ripoll
Assistant Professor
We show how to apply Sobol’s method of global sensitivity analysis to measure the influence exerted by a set of nodes’ evidence on a quantity of interest expressed by a Bayesian network. Our method exploits the network structure so as to transform the problem of Sobol index estimation into that of marginalization inference and, unlike Monte Carlo based estimators for variance-based sensitivity analysis, it gives exact results when exact inference is used. Moreover, the method supports the case of correlated inputs and it is efficient as long as eliminating the inputs’ ancestors is computationally affordable. The proposed algorithms are inspired by the field of tensor networks and generalize earlier tensor sensitivity techniques from the acyclic to the cyclic case. We demonstrate our method on three medium to large Bayesian networks in the areas of structural reliability and project risk management.
Assistant Professor
Assistant Professor
INQUIRY -