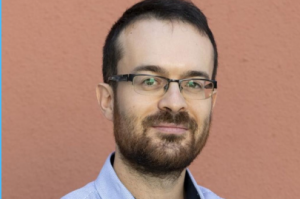
Rafael Ballester-Ripoll
Assistant Professor
Insightful visualization of multidimensional scalar fields, in particular parameter spaces, is key to many computational science and engineering disciplines. We propose a principal component-based approach to visualize such fields that accurately reflects their sensitivity to their input parameters. The method performs dimensionality reduction on the space formed by all possible partial functions (i.e., those defined by fixing one or more input parameters to specific values), which are projected to low-dimensional parameterized manifolds such as 3D curves, surfaces, and ensembles thereof. Our mapping provides a direct geometrical and visual interpretation in terms of Sobol’s celebrated method for variance-based sensitivity analysis. We furthermore contribute a practical realization of the proposed method by means of tensor decomposition, which enables accurate yet interactive integration and multilinear principal component analysis of high-dimensional models.
Assistant Professor
INQUIRY -