14/11/2022
The latest cohort of the Master in Business Analytics & Big Data graduated from an intense year of classes, projects and presentations. Luca Fiume, who recently completed the program, looks back on the challenges and rewards while sharing all his tips and lessons learned to inspire future students pursuing this unique, multidisciplinary program.
Prior to starting the Master in Business Analytics & Big Data, I was working in customer service and business development for a mobility startup in Madrid. I kept thinking that many of the tasks and projects could be fast-tracked by leveraging data-focused approaches. For example, new business accounts could’ve been generated more cost-effectively by developing a web-scraping solution oriented towards industry-specific blogs and repositories. Also, the data generated from the product’s web application could’ve opened up a new revenue stream by developing an API gateway to share information with other mobility actors complementing the startup’s service.
It was during this experience that I realized that my previous studies were outdated. The learning I had under my belt didn’t offer me a comprehensive vision of business analytics and big data today. I was sure the solutions that we needed existed, but I didn’t know what the right steps were to implement a data solution, nor what the best data tools were. That’s how I knew it was time to apply for the Master in Business Analytics & Big Data.
What to expect in the Master in Business Analytics & Big Data
The Master in Business Analytics & Big Data at IE University is a challenging program spanning over 10 months full-time, or 17 months on a part-time, blended schedule. I rose to the challenge and attended every class in person, which was made possible by the university’s innovative measures that ensured a safe learning space.
The program’s content spans four main areas of learning: Programming, Data Warehousing, Machine Learning and Distributed Computing. Already having knowledge in the first two, I was most looking forward to the latter two topics. The classes are very hands-on and require students to team up to present their impact projects at the end of every term.
The course structure is well organized, allowing students to home in on specific topics according to their goals and interests. After an introductory pre-program period, the first term laid the foundations for data structures, coding skills and machine learning, as well as programming related to data analysis and the tools for distributed computing. The second term focused on the specific subfields of big data, AI and machine learning methods, while delving deeper into digital analytics and data visualization. Afterward, a four-month elective period allowed students to customize the program according to their interests and choose which concentration area they’d like to specialize in.
Hard work pays off: the program’s biggest challenges
Throughout the year, tight deadlines kept the pressure high. Group coursework and team presentations were cornerstone moments as they helped us materialize and present the knowledge we acquired in the previous months of learning. Having two datathons organized by external companies acting as stakeholders made the experience much more realistic, helping us understand the challenges present in team-based data projects. We also enhanced our soft skills, which were needed to give presentations, collaborate with others and prioritize tasks.From the very start, one challenging aspect of the program was clear: integrating into a class of 30 people from different cultural, professional and educational backgrounds was going to be difficult. Some people think and work the same, while others have very unique ways of tackling a challenge—and all equipped with varied skill sets. From the get-go, it’s essential to meet as many people as possible and identify those students who complement your skill set. This becomes very important when asked to form workgroups because it allows you to create a balanced and diverse team with an equal distribution of work. Knowing who to rely on for which tasks is very powerful.
Outside the classroom, though, it’s important to enjoy the journey and remember that everyone is struggling with the same projects and deadlines.
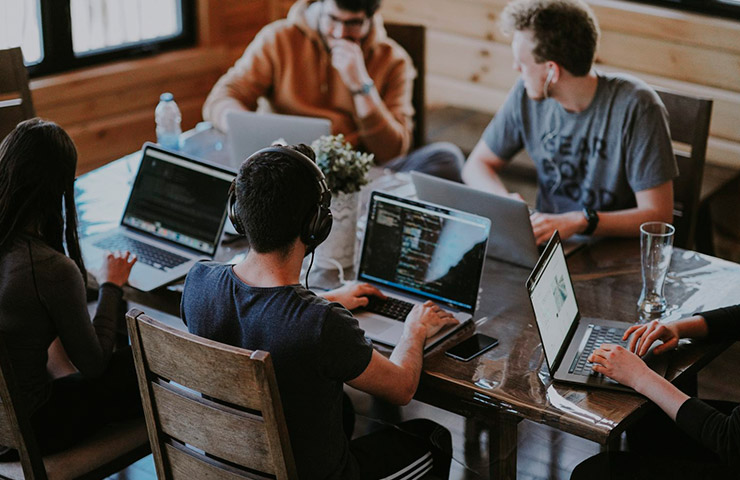
Meeting up, exchanging ideas, and asking for help always pays off. The people who have enjoyed the program the most are those that have shared the ups and downs of the master’s together, not alone.
Is the Master in Business Analytics & Big Data for me?
A very common concern for program applicants is the level of coding fluency needed to apply. Fear not! Some students come to the program without ever having coded in their lives. However, coding should be demystified as soon as possible. The pre-program is highly recommended for this, ensuring that you start the first term with some coding basics under your belt, which will help later on when the topics become more complex.
To a certain degree, this program allows you to choose your preferred programming language, between R and Python. My advice would be to choose one of the two to focus on. Knowing the basics of both is essential, but it’s important to start specializing in one or the other. This means exploring and consistently working with the same packages, building a notebook code library to reuse in ML projects and earning additional certifications from online courses for an advanced understanding of your preferred language. In the long run, this will help you gain mastery in one language and bootstrap ML models much faster.
In the end, as long as you have the motivation and curiosity to learn, the Master in Business Analytics & Big Data is suitable for any student with a varied academic background. In my group projects, I’ve been inspired by the caliber of work and dedication put in by my peers who had non-technical professional backgrounds. data projects in business contexts for years to come.
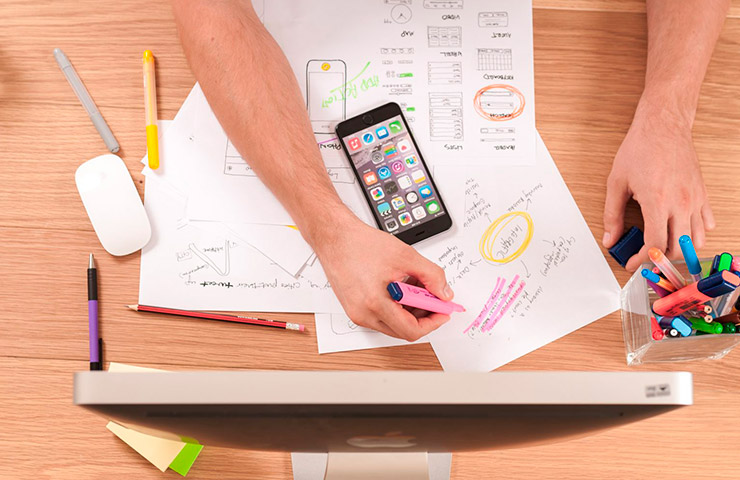
They are the ones who learned the most and will benefit the most from their multidisciplinary knowledge.
Tips and tricks for success in the program
There comes a stage in the later terms where all these individual subjects start to make sense in relation to one another. It’s a sort of “eureka” moment where all the topics start fitting together as a cohesive ensemble of tools to obtain a final business objective. This is the best moment to take the time to try and understand the bigger picture. Machine learning and distributed computing are two different fields of study but when merged, they bring the biggest impact to business. Obtaining this general perspective also helps you to know yourself better because it’ll allow you to better understand what kind of role you’d like to fill in the data world.
When you get towards the end of the program and start applying for positions, always research the companies, industries and job roles well in advance. The IE Talent & Careers team is very helpful in this process: they offer students the opportunity to first choose a career path and then tailor their job hunt accordingly. There are very specific certifications, skills and interview processes depending on each career path chosen. Selecting one career path as soon as possible allows you to better prepare and ultimately increase your chances of finding a job you love.
Final takeaways
I’m very proud of myself for everything I’ve achieved in this tough and fast-paced program. I’ve learned and applied concepts in machine learning and computer vision that seemed so academic and well above my means to understand just 10 months ago. It’s very empowering to come out of the program and look back at the innumerable coding notebooks, visualization dashboards, and presentations that we delivered. The road ahead to data mastery is still long, but starting my data career with the Master in Business Analytics & Big Data has given me the tools and confidence to deliver data projects in business contexts for years to come.